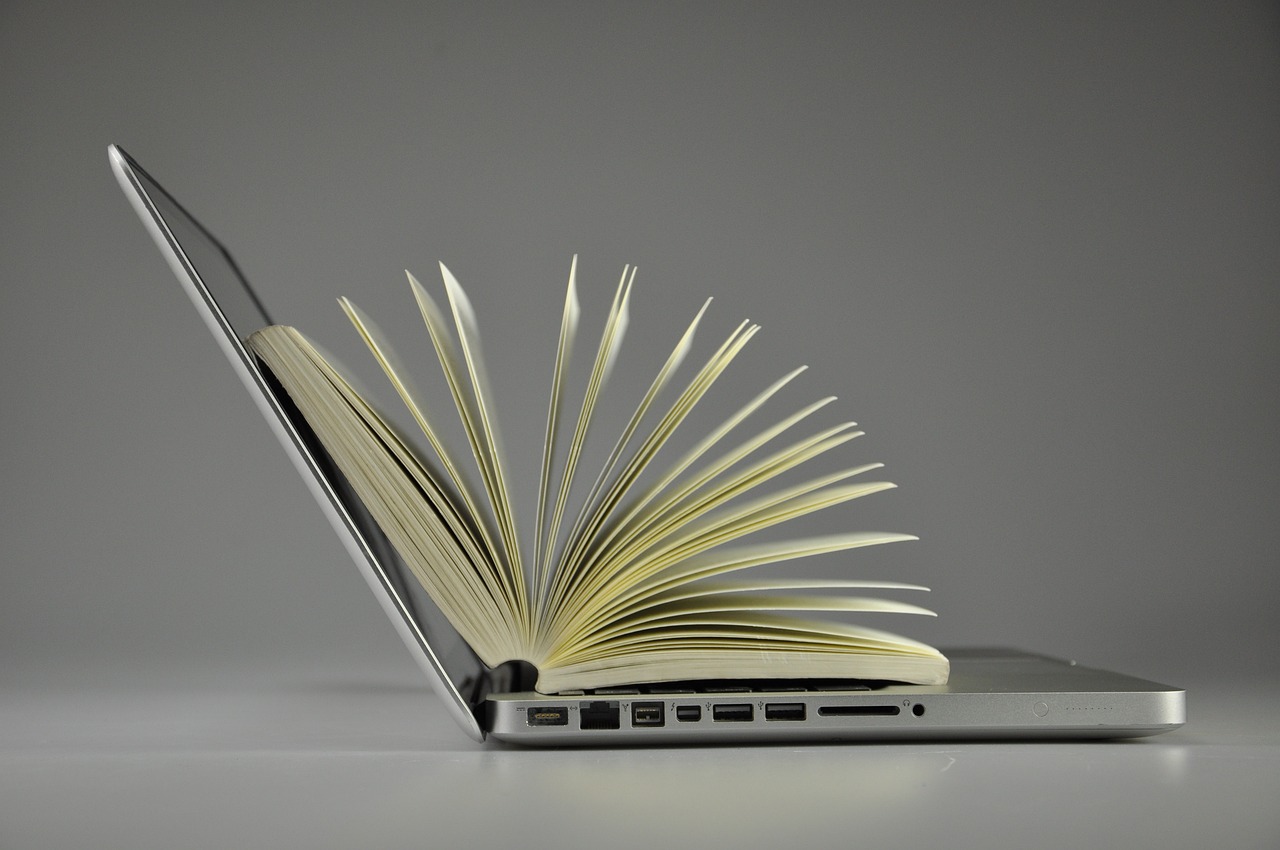

Key Takeaways:
- Unstructured data from sources like clinical notes can provide valuable real-world insights to augment structured clinical trial data in drug development.
- Natural language processing (NLP) enables mining of unstructured text data for information on drug efficacy, side effects, patient behaviors, and more.
- Challenges include data privacy, integration across sources, and developing reliable NLP models to extract accurate insights.
- Proper governance and cross-functional collaboration is needed to safely and effectively leverage unstructured data.
- Responsible use of unstructured notes has the potential to accelerate drug development, improve safety monitoring, and support value-based care models.
The Untapped Potential of Unstructured Data
In the meticulous world of drug development, every data point is precious. Clinical trials generate a wealth of rigorously structured efficacy and safety data. During routine clinical care, computerized physician order entry systems and electronic health records capture structured data that can enhance drug efficacy and safety monitoring. However, an underutilized treasure trove of real-world information exists in the unstructured text of clinical notes, hospital records, and other loosely formatted sources gathered as part of standard medical practice.
Unleashing Insights with Natural Language Processing
Historically, this unstructured data has been difficult to integrate and analyze alongside its structured counterparts. This is partly due to variability in documentation practices among different healthcare providers. Additionally, the extraction of relevant data has traditionally relied on manual review and interpretation by clinically trained personnel. But major pharmaceutical companies are now investing heavily in natural language processing (NLP) to mine these unstructured sources for insights.
While NLP does not eliminate the need for human involvement, it can significantly streamline the process. NLP serves as a tool that works in conjunction with human interaction, combining the efficiency of intelligent automation with the ability to incorporate human feedback. This combination allows for more effective extraction of insights from unstructured data, which ultimately aids in accelerating research, optimizing clinical trials, and enhancing drug safety monitoring.
Applications: From Safety Signals to Patient Experiences
So when and how can unstructured data provide value? One key application is using NLP models trained on doctors’ notes to identify potential safety signals that may not surface until after a drug is approved and prescribed at scale. These real-world signals can prompt further investigations and narrow the “surrogate to reality” gap between clinical trials and clinical practice.
Unstructured data has also shown promise in two critical areas: better defining appropriate inclusion/exclusion criteria for clinical trials and identifying under-represented patient populations who may benefit from a treatment. By processing clinical records from diverse practices, researchers can find more suitable study cohorts for targeted recruitment efforts to ensure that clinical trials are more representative of real-world patient populations. Furthermore, analyzing unstructured data allows for a better understanding of real-world behaviors like treatment adherence or self-reporting of side effects.
Another valuable application of NLP is in tracking and codifying patients’ experiences based on anecdotal descriptions found in clinical notes. For example, phrases such as “The medicine made me feel queasy” can provide qualitative context around drug effects and quality-of-life. This context could support reporting requirements for post-marketing adverse events. Additionally, other qualitative context could complement clinical scoring tools used in the trial setting, potentially expediting label expansions for new indications.
Overcoming Obstacles to Implementation
Despite the opportunities, integrating unstructured data is not without challenges. Concerns around patient privacy and data security pose hurdles. While unstructured text provided for research purposes is typically de-identified, residual identifying information can remain. Utilizing data sources that have gone through multiple layers of de-identification efforts is crucial to mitigate this risk effectively. Further, reliably extracting structured insights from unstructured text across multiple source systems using NLP also presents difficulties.
Developing robust, production-grade NLP models requires immense training data, careful tuning to the healthcare/biomedical domain, and systematic quality testing. Merging unstructured insights with existing structured pipelines is also an intricate systems engineering challenge.
Data Governance and the Path Forward
Looking ahead, stakeholders agree that managed responsibly and embedded into robust data frameworks, unstructured real-world datasets can help drive high quality healthcare to its full potential. Pharmaceutical companies may find accelerated paths to drug approvals and label expansions. Payers could gain transparency to optimize formularies and pricing models. And ultimately, patients may benefit from better targeted treatments.
Sources:
- “Unlocking the Power of Unstructured Data in Drug Discovery.” DrugDiscoveryToday, 2021..
- “Natural Language Processing in Drug Safety.” Pharmaceutical Medicine, 2021.
- “Bridging the ‘Efficacy-to-Effectiveness’ Gap.” BioPharmaDive, 2022.
- “NLP for Clinical Trial Eligibility Criteria.” NEJM Catalyst, 2021.
- “NLP Applications in Life Sciences and Healthcare.” Optum, 2022.
- “Challenges of Integrating Unstructured Data in Healthcare.” MIT, 2020.
- “Data, RWE and the Future of Value-Based Care.” IQVIA, 2022.