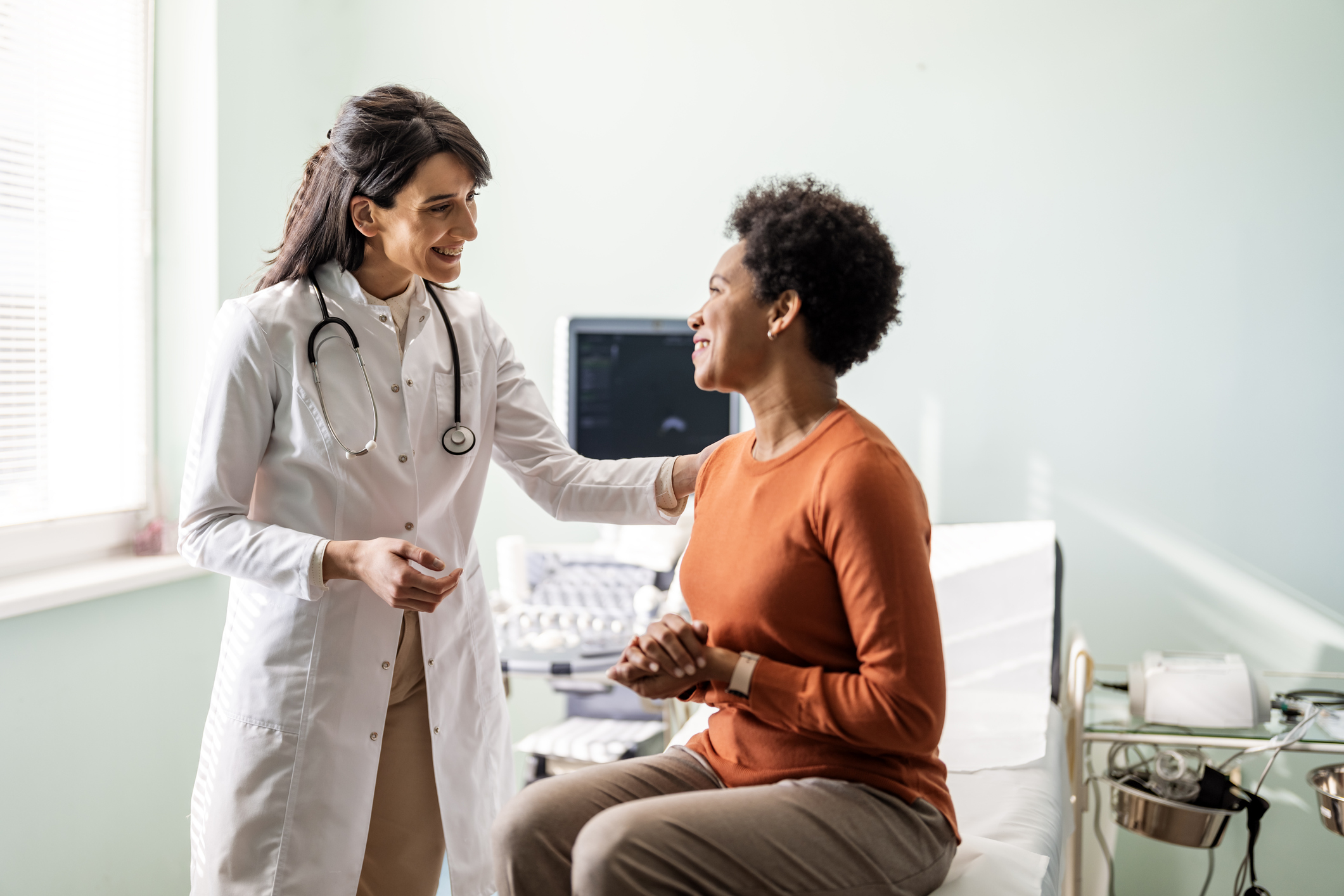
Key Takeaways:
- The US has a primarily private, market-based healthcare system, while most European countries have universal, tax-funded public healthcare systems.
- Healthcare spending per capita is substantially higher in the US compared to Europe, yet the US lags behind on metrics like life expectancy and infant mortality.
- European healthcare systems aim to provide comprehensive coverage to all citizens, while millions remain uninsured in the US despite the Affordable Care Act.
- Wait times for non-urgent care tend to be longer in European public systems, though the US has longer wait times for emergency room visits.
- The US healthcare system is dominated by for-profit providers and private insurance companies, leading to higher costs but more cutting-edge treatments.
The Opposing Models
For decades, the healthcare systems of the United States and Europe have taken vastly different approaches, sparking heated debate over which model delivers better care at a sustainable cost. While the US relies primarily on private insurance and market competition, most European nations have adopted tax-funded universal healthcare as a basic right for all citizens.
Aspect | United States | Europe |
System Type | Private, market-based healthcare system. | Universal, tax-funded public healthcare systems. |
Healthcare Spending | Substantially higher per capita compared to Europe. 2019: $11,072 per person. | Lower per capita compared to the US. Average across wealthy European nations in 2019: $5,505. |
Health Outcomes | Lags behind Europe on metrics like life expectancy and infant mortality. | Better life expectancy and lower infant mortality rates compared to the US. |
Coverage | Millions remain uninsured despite the Affordable Care Act. Approximately 28 million non-elderly Americans are uninsured. | Comprehensive coverage for all citizens. Nearly universal coverage with little to no out-of-pocket costs for services. |
Provider System | Dominated by for-profit providers and private insurance companies. | Predominantly public-funded healthcare systems, with some countries incorporating a mix of private and public insurance schemes. |
Wait Times | Longer wait times for emergency room visits. More efficient access to specialized treatments and newly approved therapies. | Longer wait times for non-urgent care in public systems due to budget and capacity management. Generally quicker access to emergency services. |
Innovation and Costs | High healthcare spending contributes to cutting-edge medical innovations. Costs driven up by profit motives, administrative complexities, and fee-for-service model. | Emphasizes cost controls, standardized fee schedules, and integrated care delivery. While innovative, may have slower access to some new treatments due to budget considerations. |
Future Challenges | Aging population, rising chronic disease burden, and debates over the extent of government involvement. Calls for “Medicare for All” reflect ongoing debates. | Similar challenges with aging populations and chronic diseases. Experiments with public-private hybrid schemes and value-based reimbursements to maintain sustainability. |
Philosophical Approach | Healthcare often viewed as a market commodity, with ongoing debates about its status as a human right. | Healthcare generally considered a basic right for all citizens, funded through taxation. |
US: Private Markets vs. Europe: Public Coverage
At its core, the American healthcare system is a private, decentralized collection of for-profit hospitals, clinics, insurance companies, and other providers incentivized to maximize revenues. Employers typically offer private insurance plans, and government programs like Medicare and Medicaid cover the elderly and low-income populations. However, an estimated 28 million non-elderly Americans remain uninsured despite the Affordable Care Act’s coverage expansions.
In stark contrast, European healthcare systems are overwhelmingly publicly-funded through taxation to ensure universal coverage for all legal residents. Countries like the UK, Spain, and Sweden operate single-payer national health services, while others like Germany, France, and the Netherlands have multi-payer universal systems that incorporate a mix of private and public insurance schemes.
Higher Costs, Lagging Outcomes in the US
One indisputable fact is that the US healthcare system is exorbitantly more expensive per capita than any European model, yet its health outcomes lag behind on metrics like life expectancy and infant mortality. In 2019, US healthcare spending reached $11,072 per person – over double the average of $5,505 across wealthy European nations.
Many experts attribute the US system’s high costs to profit-driven incentives, administrative complexities associated with private insurers, and a fee-for-service payment structure that encourages more treatment over quality outcomes. European systems emphasize cost controls, standardized fee schedules, and integrated care delivery as more efficient alternatives.
The Trade-Off: Innovation vs. Wait Times
However, America’s high healthcare spending does contribute to cutting-edge medical innovations and reduced wait times for specialized treatments like newly approved cancer therapies. European systems often face longer delays for non-urgent services as public health authorities aim to manage finite budgets and capacity. Although the US has lengthier emergency room wait times on average than European countries.
Universal Coverage vs. Uninsured Millions
Access to healthcare also differs significantly between the US and Europe. The Affordable Care Act brought the US’s uninsured rate below 10% for the first time, but millions still lack coverage and face potentially bankrupting medical bills. European universal systems cover all citizens cradle-to-grave – services like preventative screenings, hospital stays, specialist visits, surgeries, prescribed medications, and prenatal care are fully covered with little or no out-of-pocket costs beyond modest copays.
Challenges for the Future
Both systems face mounting challenges from aging populations and rising costs associated with chronic diseases and new technologies. European nations are experimenting with public-private hybrid schemes and value-based reimbursements, while American policymakers continue debating the role of government involvement amidst calls for “Medicare for All.”
Differing Philosophies
Ultimately, the US and Europe represent vastly different philosophical and economic approaches to the simple question: Should healthcare be considered a human right or a market commodity? The answer, and the path forward, remains highly contentious for two world powers with no easy solutions in sight.
Sources:
- “U.S. Health Care Resources.” American Hospital Association, 2021.
- “Health Insurance Coverage in the United States.” Centers for Disease Control and Prevention, 2022.
- “Health Systems Characteristics.” OECD Health Statistics, 2021.
- “Health Care Systems in the EU.” European Union, 2021.
- “How Does the Quality of the U.S. Health-Care System Compare to Other Countries?” Peterson-KFF Health System Tracker, 2022.
- “Health Expenditure Per Capita.” OECD Health Statistics, 2022.
- Sawyer, B., et al. “Why Do Health Care Costs Keep Rising?” Peterson-KFF, 2022.
- “The United States Leads Rising Availability of Cancer Medicines.” IQVIA, 2021.
- “Waiting Times for Health Services Next?” EuroHealth, 2020.
- “U.S. Emergency Department Visit Data Visualizations.” CDC, 2018.
- “Universal Health Coverage.” World Health Organization, 2021.
- “Value-Based Healthcare in Europe.” EIT Health, 2022.