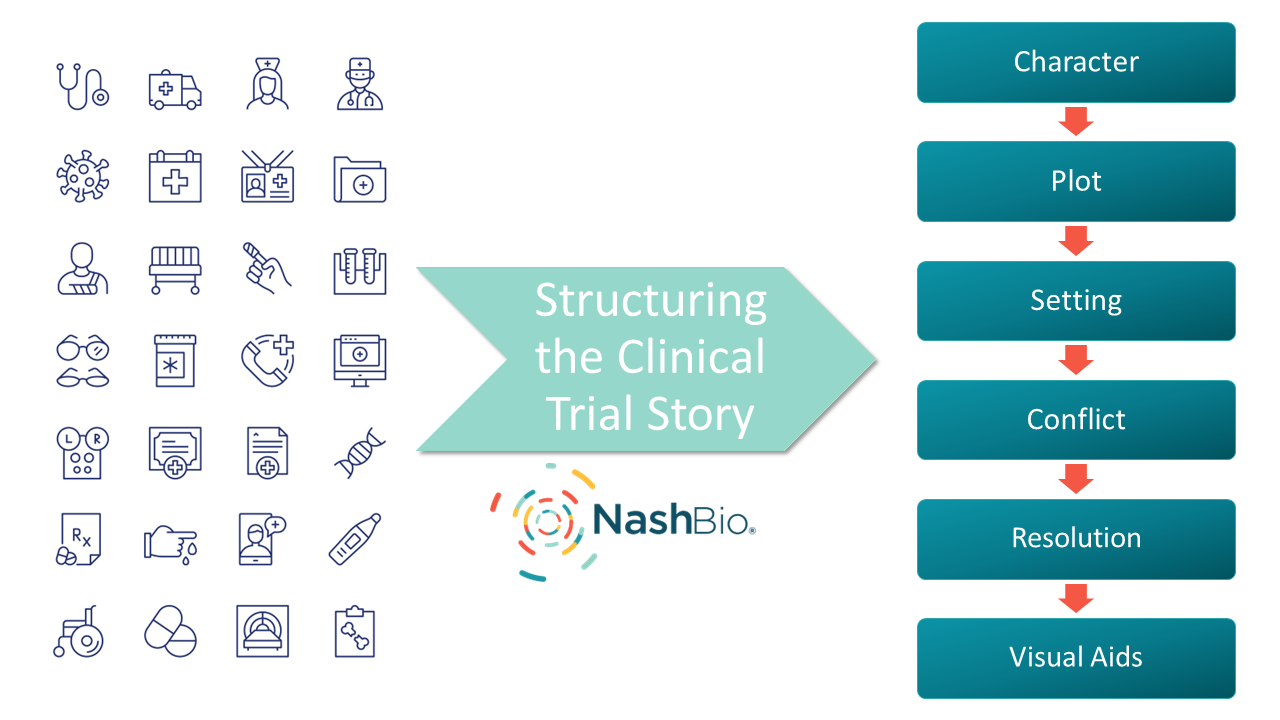

Key Takeaways:
- Integrating elements of storytelling enriches the presentation of clinical trial data, making it more engaging and informative.
- Analyzing raw clinical trial data reveals hidden trends and patterns upon which to build a compelling story.
- Adhering to ethical and regulatory standards is imperative when crafting narratives from clinical trial findings.
- Augmenting clinical trial data with real-world outcomes allows for a more comprehensive understanding of treatment effectiveness, representing diverse demographics and lifestyles often underrepresented in the controlled clinical trial environment.
- Communicating the real-world impact of treatments beyond statistical outcomes is crucial for showcasing scientific advancement to a broad audience.
In pharmaceutical research and development, the path from clinical trials to market is strictly defined. It involves analyzing data, conducting research, and ensuring compliance with regulations. It is essential to follow these steps to ensure the safety and efficacy of the drug. Although clinical trial data are often presented scientifically, they contain the potential for powerful storytelling beyond statistical tables and regulatory filings.
In this blog post, we explore the art of storytelling with clinical trial data and discuss how real-world perspectives can augment these insights.
Structuring the Story
Great storytelling hinges on certain fundamental elements. Below are a few of these elements and ways each can be applied to clinical trial data to help inform and engage the audience.
- Character. In clinical trials, “character” refers to the intervention under study. Highlight the features, benefits, and potential impacts of the intervention.
- Plot. Create a clear storyline. Start with the problem (condition or disease), introduce an intervention (treatment), describe the process (methodology), and conclude with the results.
- Setting. Provide context by explaining the background and significance of the studied condition and why the clinical trial is crucial for advancing treatment.
- Conflict. Discuss challenges faced during the clinical trial, such as recruitment difficulties or unexpected side effects.
- Resolution. Share the clinical trial outcomes. Present statistical results, efficacy, safety, and any breakthrough findings.
- Visual aids. Incorporate visuals such as infographics, charts, and interactive dashboards to make complex information more accessible and engaging.
Uncovering the Story
Interpreting clinical trial results can be challenging. To ensure findings are accessible to a broad audience, it is important to construct a compelling narrative around the data.
Clinical trial data serve as the backbone of drug development. In raw form, these data include patient demographics, treatment protocols, treatment responses, adverse events, and efficacy outcomes presented as numbers, tables, and graphs. However, analyses are often required to help uncover the stories within clinical trial data.
Analyzing clinical trial data allows for realizing trends and patterns that are not immediately apparent. Whether it’s the impact of a specific treatment on a particular subgroup or long-term effects beyond the clinical trial period, these insights contribute to a richer and more comprehensive storyline.
Beyond numerical outcomes and statistical significance, the impact of treatment extends to its ability to address unmet medical needs and improve patient outcomes. A balanced presentation of risks and benefits empowers healthcare professionals, policymakers, and patients to understand the data’s implications and make conscientious decisions.
Maintaining ethical standards and transparency is crucial to this process. Adhering to regulatory guidelines and clearly articulating limitations and biases ensures integrity in storytelling with clinical trial data.
Supporting the Story
Clinical trials are only one phase of a drug development lifecycle. Understanding the long-term safety and effectiveness of a treatment often requires evaluation beyond the controlled clinical trial environment.
For example, supplementing clinical trial data with real-world outcomes helps validate hypotheses, identify potential biomarkers, and uncover post-marketing insights. This approach accommodates variations in demographics, socioeconomics, and lifestyle choices often excluded from controlled clinical trials.
In addition, communicating how a treatment translates into improved quality of life or reduced healthcare burden further solidifies the narrative.
Summary
Clinical trial data are critical for advancing healthcare but are often challenging to interpret. Transforming clinical trial data into compelling stories involves focusing on the character, plot, setting, conflict, and resolution of the study while enriching the data with real-world perspectives. Crafting narratives that resonate with diverse stakeholders is crucial for conveying the true impact of treatments driving research, and advancing healthcare for the benefit of everyone.