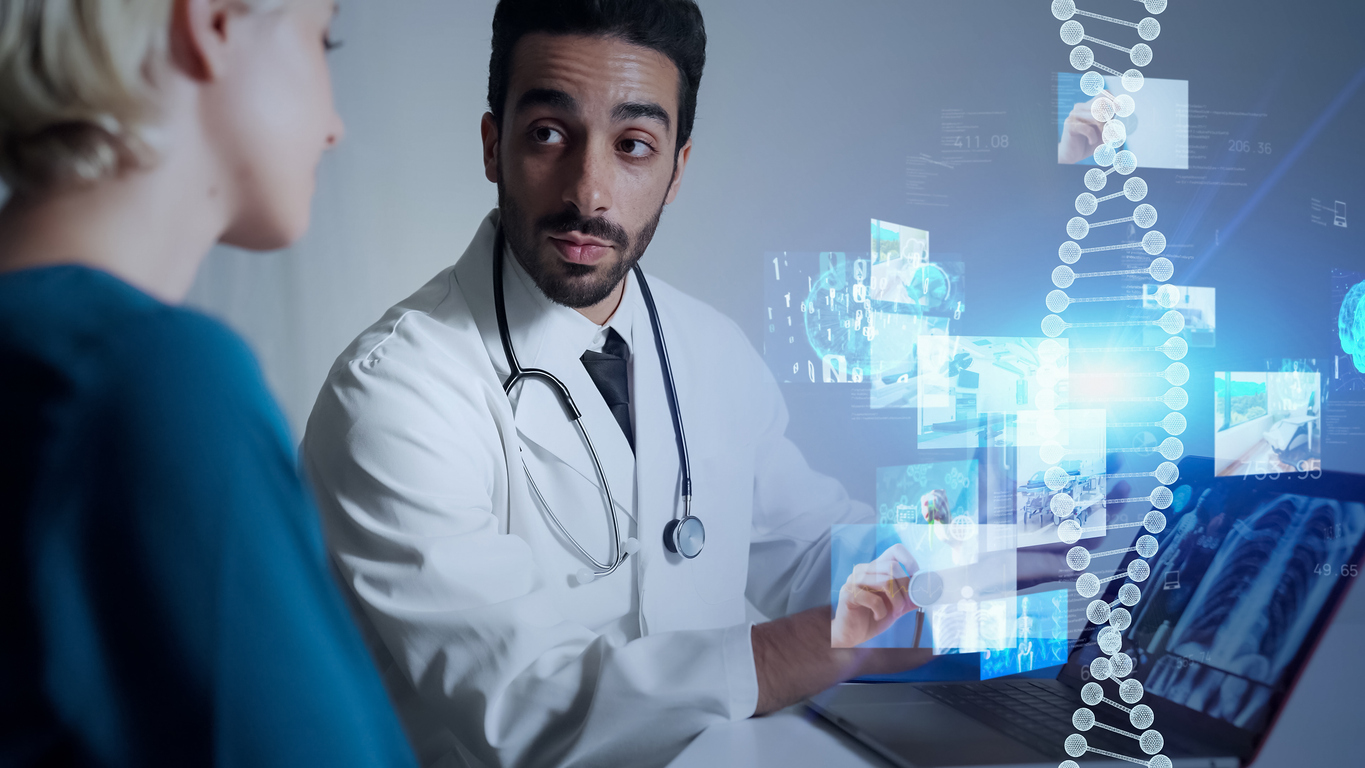
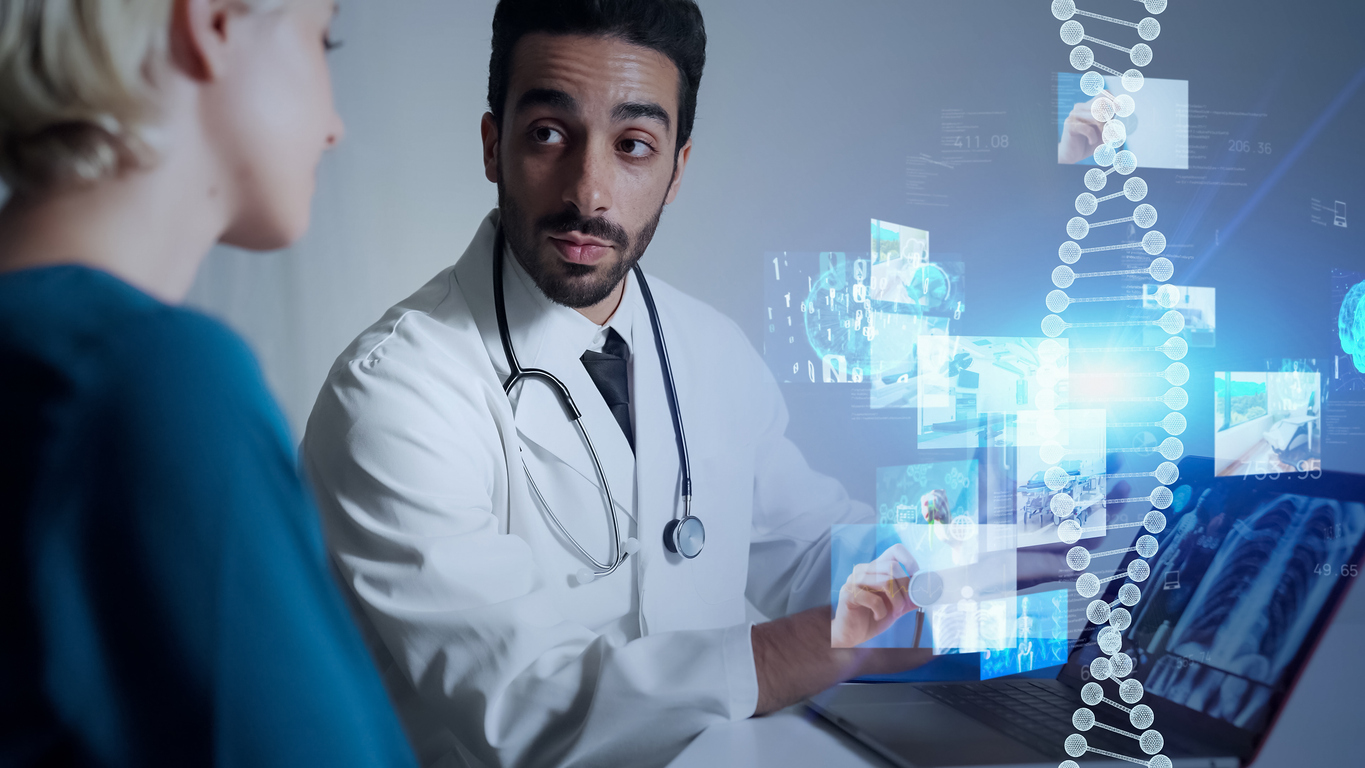
3841 Green Hills Village Dr., Suite 200
Nashville, TN 37215-2691
4660 La Jolla Village Drive
Suites 100 & 200
San Diego, California 92122
(615) 208-5462
info@nashbio.com
3841 Green Hills Village Dr., Suite 200
Nashville, TN 37215-2691
4660 La Jolla Village Drive
Suites 100 & 200
San Diego, California 92122
(615) 208-5462
info@nashbio.com
© 2024 Nashville Biosciences, LLC. All Rights Reserved. | Leveraging Vanderbilt University Innovation
© 2024 Nashville Biosciences, LLC. All Rights Reserved. | Leveraging Vanderbilt University Innovation
© 2024 Nashville Biosciences.
Genomics is one of the fastest developing research areas in the world today. Due to rapid advancements, in-depth genetic analysis has become essential in R&D and clinical practice.
With drug development costs forecast to double every nine years, we need more than ever to improve R&D productivity and uncover new ways to diagnose and care for diseases more efficiently. In order to increase the speed-to-market for life sciences companies, it’s imperative that we fully recognize and capture the potential of human genetics in drug and diagnostics R&D.