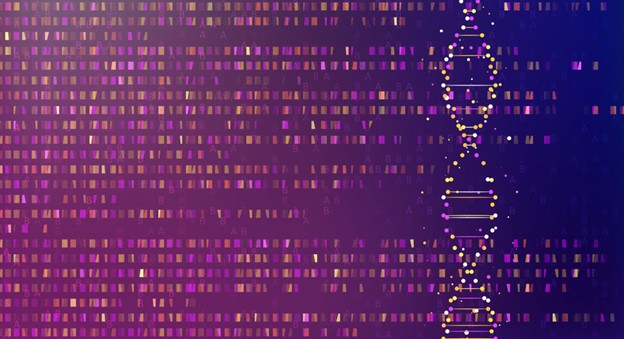
Introduction
We were promised the end to genetic diseases. All we needed to do was unlock the human genome. Unfortunately, life has a way of being more complicated than we expect. It turned out that many genetic disorders are the result of the interplay between multiple genetic factors. This set off the need for improved analytical tools to analyze human genetics that could interrogate the associations of many genetic backgrounds and link them to various diseases. One such technique, the Polygenic Risk Score (PRS), emerged as a powerful tool to quantify the cumulative effects of multiple genetic variants on an individual’s predisposition to a specific disease.
The Evolution of Polygenic Risk Scores
The genesis of PRS can be traced back to the early 2000s when researchers sought to comprehend the collective impact of multiple genetic variants on disease susceptibility. Initially viewed through a biological lens, the focus was on enhancing the prediction of diseases by analyzing subtle genomic variations. Studies concentrated on prevalent yet complex diseases such as diabetes, cardiovascular diseases, and cancer, laying the groundwork for a comprehensive understanding of their genetic architecture.
That was until Dr. Sekar Kathiresan showed that the prediction from a PRS was just as clinically useful as a single variant (Khera et al., 2018). Instead of looking at the percent of people with a PRS in each group (with or without a disease), his group could show a much more obvious effect – the difference in risk for people in the groups with the highest and lowest scores. Then, they could say that there was a huge difference in risk for these two edges of the population.
In the initial stages, PRSs consisted of only the most statistically significant variants from genome-wide association studies. Geneticists often added up the quantity of risk variants without giving them a weight for how much of an impact they had on whether someone would get a disease. Refining these scores led scientists to challenge arbitrary risk cutoffs and advocate for the inclusion of all variants to maximize statistical power (based on the assumption that, on average, variants that have no effect are evenly distributed to appear positively or negatively correlated to the trait). However, proximity of variants on a chromosome presented another challenge. If variants were closer together on a chromosome, they would be less likely to be separated during recombination (Linkage Disequilibrium). This would result in them carrying the signal of something that had a true effect, potentially leading to an overcounting of that signal.
To deal with this, geneticists used tools to remove signals within a specified block unless their correlation with the strongest signal fell below a threshold. One of the first packages, PRSice (Choi & O’Reilly, 2019), used an approach called Pruning and Thresholding. Scientists would choose a block size, say, 200,000 base pairs. A program would go through and slide that block along the genome. If there was more than a single signal in that block, the program would remove (or “prune”) all but the strongest signal unless the variant had a smaller correlation with the strongest signal than the “threshold”. The result was that in a region with many different variants that affected the risk of a disease, but which were still a bit correlated, signal could be lost.
Criticism from biostatisticians prompted a shift towards a Bayesian approach, reducing over-counting while better accounting for partially independent signals. Implementation was challenged by the extensive computational resources needed to update the signal at each genetic location based on linkage disequilibrium of the surrounding SNPs. One program, called PRS-CS (Ge et al., 2019), implemented a method that could apply changes to a whole linkage block at once, addressing both the geneticist demand for a good system that can provide results using the computation tools we have and the biostatistician demand for accuracy and retained information.
Despite these advancements, accuracy challenges persisted, particularly when applying scoring systems across populations with different genetic ancestries. It turned out Linkage Disequilibrium was a pervasive problem. The patterns of Linkage Disequilibrium are different in people with different genetic ancestries. In fact, even statistics about the patterns themselves, like how big an average block size is, are different. Recognizing the need for improvement, ongoing efforts in refining PRSs aim to address these challenges, paving the way for more accurate and reliable applications. As researchers delve deeper into these complexities, the evolving landscape of PRSs continues to shape the future of clinical research.
Polygenic Risk Scores in Clinical Research Settings
To harness the full potential of PRS in clinical practice, a crucial shift is needed—from population-level insights to personalized predictions for individual patients. This transformation involves converting relative risks, which compare individuals across the PRS spectrum with a baseline group, into absolute risks for the specific disease (Lewis & Vassos, 2020). The current emphasis is on identifying individuals with a high genetic predisposition to disease, forming the foundation for effective risk stratification. This information guides decisions related to participation in screening programs, lifestyle modifications, or preventive treatments when deemed suitable.
In practical applications, PRS demonstrates promise in patient populations with a high likelihood of disease. Consider a recent study in an East Asian population, where researchers developed a PRS for Coronary Artery Disease (CAD) using 540 genetic variants (Lu et al., 2022). Tested on 41,271 individuals, the top 20% had a three-fold higher risk of CAD compared to the bottom 20%, with lifetime risks of 15.9% and 5.8%, respectively. Adding PRS to clinical risk assessment slightly improved accuracy. Notably, individuals with intermediate clinical risk and high PRS reached risk levels similar to high clinical risk individuals with intermediate PRS, indicating the potential of PRS to refine risk assessment and identify those requiring targeted interventions for CAD.
Another application of PRS lies in improving screening for individuals with major disease risk alleles (Roberts et al., 2023). A recent breast cancer risk assessment study explored pathogenic variants in high and moderate-risk genes (Gao et al., 2021). Over 95% of BRCA1, BRCA2, and PALB2 carriers had a lifetime breast cancer risk exceeding 20%. Conversely, integrating PRS identified over 30% of CHEK2 and almost half of ATM carriers below the 20% threshold. Indeed, a similar result was found in a separate study when researchers investigated men with high blood levels of prostate-specific antigen (PSA).
This trend extends to other diseases, such as prostate cancer, where a separate investigation focused on men with elevated levels of prostate-specific antigen (PSA) (Shi et al., 2023). Through the application of PRS, researchers pinpointed over 100 genetic variations linked to increased PSA levels. Ordinarily, such elevated PSA levels would prompt prostate biopsies to assess potential prostate cancer. By incorporating PRS into the screening process, doctors could have accounted for the natural variation in PSA level and prevent unnecessary escalation of clinical care. These two studies suggest that PRS integration into health screening enhances accuracy, preventing unnecessary tests and enabling more personalized risk management.
In the realm of pharmacogenetics, efforts to optimize treatment responses continue. While progress has been made in identifying rare high-risk variants linked to adverse drug events, predicting treatment effectiveness remains challenging. The evolving role of PRS in treatment response is particularly evident in statin use for reducing initial coronary events. In a real-world cohort without prior myocardial infarction, an investigation revealed that statin effectiveness varied based on CHD PRSs, with the highest impact in the high-risk group, intermediate in the intermediate-risk group, and the smallest effect in the low-risk group (Oni-Orisan et al., 2022). Post-hoc analyses like this for therapeutics could potentially allow for more targeted enrollment for clinical trial design, substantially reducing the number of participants needed to demonstrate trial efficacy (Fahed et al., 2022).
Conclusion
As the field of genetics continues to advance, PRSs emerge as a potent tool with the potential to aid clinical research. Validated PRSs show promise in enhancing the design and execution of clinical trials, refining disease screening, and developing personalized treatment strategies to improve the overall health and well-being of patients. However, it’s crucial to acknowledge that the majority of PRS studies heavily rely on biased datasets of European ancestry. To refine and improve PRS, a comprehensive understanding of population genetic traits for people of all backgrounds, such as linkage disequilibrium, is essential. Moving forward, the integration of PRS into clinical applications must prioritize datasets with diverse ancestry to ensure equitable and effective utilization across all patient backgrounds. As research in this field progresses, the incorporation of PRS is poised to become an indispensable tool for expediting the development of safer and more efficacious therapeutics.
References
Choi, S. W., & O’Reilly, P. F. (2019). PRSice-2: Polygenic Risk Score software for biobank-scale data. GigaScience, 8(7). https://doi.org/10.1093/gigascience/giz082
Fahed, A. C., Philippakis, A. A., & Khera, A. V. (2022). The potential of polygenic scores to improve cost and efficiency of clinical trials. Nature Communications, 13(1), 2922. https://doi.org/10.1038/s41467-022-30675-z
Gao, C., Polley, E. C., Hart, S. N., Huang, H., Hu, C., Gnanaolivu, R., Lilyquist, J., Boddicker, N. J., Na, J., Ambrosone, C. B., Auer, P. L., Bernstein, L., Burnside, E. S., Eliassen, A. H., Gaudet, M. M., Haiman, C., Hunter, D. J., Jacobs, E. J., John, E. M., … Kraft, P. (2021). Risk of Breast Cancer Among Carriers of Pathogenic Variants in Breast Cancer Predisposition Genes Varies by Polygenic Risk Score. Journal of Clinical Oncology : Official Journal of the American Society of Clinical Oncology, 39(23), 2564–2573. https://doi.org/10.1200/JCO.20.01992
Ge, T., Chen, C.-Y., Ni, Y., Feng, Y.-C. A., & Smoller, J. W. (2019). Polygenic prediction via Bayesian regression and continuous shrinkage priors. Nature Communications, 10(1), 1776. https://doi.org/10.1038/s41467-019-09718-5
Khera, A. V., Chaffin, M., Aragam, K. G., Haas, M. E., Roselli, C., Choi, S. H., Natarajan, P., Lander, E. S., Lubitz, S. A., Ellinor, P. T., & Kathiresan, S. (2018). Genome-wide polygenic scores for common diseases identify individuals with risk equivalent to monogenic mutations. Nature Genetics, 50(9), 1219–1224. https://doi.org/10.1038/s41588-018-0183-z
Lewis, C. M., & Vassos, E. (2020). Polygenic risk scores: from research tools to clinical instruments. Genome Medicine, 12(1), 44. https://doi.org/10.1186/s13073-020-00742-5
Lu, X., Liu, Z., Cui, Q., Liu, F., Li, J., Niu, X., Shen, C., Hu, D., Huang, K., Chen, J., Xing, X., Zhao, Y., Lu, F., Liu, X., Cao, J., Chen, S., Ma, H., Yu, L., Wu, X., … Gu, D. (2022). A polygenic risk score improves risk stratification of coronary artery disease: a large-scale prospective Chinese cohort study. European Heart Journal, 43(18), 1702–1711. https://doi.org/10.1093/eurheartj/ehac093
Oni-Orisan, A., Haldar, T., Cayabyab, M. A. S., Ranatunga, D. K., Hoffmann, T. J., Iribarren, C., Krauss, R. M., & Risch, N. (2022). Polygenic Risk Score and Statin Relative Risk Reduction for Primary Prevention of Myocardial Infarction in a Real-World Population. Clinical Pharmacology and Therapeutics, 112(5), 1070–1078. https://doi.org/10.1002/cpt.2715
Roberts, E., Howell, S., & Evans, D. G. (2023). Polygenic risk scores and breast cancer risk prediction. Breast (Edinburgh, Scotland), 67, 71–77. https://doi.org/10.1016/j.breast.2023.01.003
Shi, M., Shelley, J. P., Schaffer, K. R., Tosoian, J. J., Bagheri, M., Witte, J. S., Kachuri, L., & Mosley, J. D. (2023). Clinical consequences of a genetic predisposition toward higher benign prostate-specific antigen levels. EBioMedicine, 97, 104838. https://doi.org/10.1016/j.ebiom.2023.104838