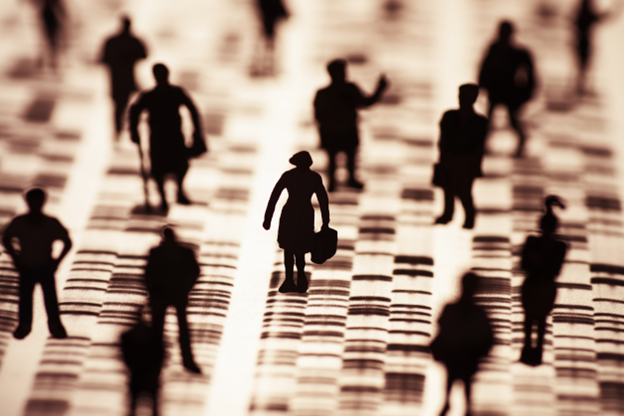
Key Takeaways:
- Polygenic risk scores aggregate the effects of thousands of genetic variants to estimate an individual’s inherited risk for complex diseases.
- Polygenic risk is based on genome-wide association studies that identify common variants associated with modest increases in disease risk.
- Polygenic scores provide risk stratification beyond family history, but most disease risk is not yet explained by known variants.
- Clinical validity and utility of polygenic scores will improve as more disease-associated variants are discovered through large genomic studies.
- Polygenic risk models may one day guide targeted screening and preventive interventions, but face challenges related to clinical interpretation and implementation.
Introduction to Polygenic Risk Scores
The vast majority of common, chronic diseases do not follow simple Mendelian inheritance patterns, but rather are complex genetic conditions arising from the combined small effects of thousands of genetic variations interacting with lifestyle and environmental factors. Polygenic risk scores aggregate information across an individual’s genome to estimate their inherited susceptibility for developing complex diseases like heart disease, cancer, diabetes and neuropsychiatric disorders.
Polygenic risk scores are constructed using data from genome-wide association studies (GWAS) that scan markers across the genomes of thousands to millions of individuals to identify genetic variants associated with specific disease outcomes. While most disease-associated variants have very small individual effects, the combined effect of thousands of these common, single nucleotide polymorphisms (SNPs) can stratify disease risk in a polygenic model.
Polygenic Scores vs. Single Gene Mutations
In monogenic diseases like cystic fibrosis and Huntington’s disease, a single genetic variant is necessary and sufficient to cause disease. Genetic testing for causal mutations in specific disease-linked genes provides a clear-cut diagnostic assessment. In contrast, no single gene variant accounts for more than a tiny fraction of risk for complex common diseases. Polygenic risk models aggregate the effects of disease-associated variants across the genome, each imparting a very modest increase or decrease in risk. An individual’s polygenic risk score reflects the cumulative impact of thousands of small risk effects spread across their genome.
While polygenic scores are probabilistic and estimate only inherited genetic susceptibility, monogenic mutations convey deterministic information about disease occurrence. However, for many individuals with elevated polygenic risk scores, modifiable lifestyle and environmental factors may outweigh their inherited predisposition, allowing prevention through early intervention.
GWAS and Polygenic Scores
Human genome-wide association studies utilize DNA microarray ‘chips’ containing hundreds of thousands to millions of SNPs across the genome. Comparing SNP frequencies between thousands of disease cases and controls reveals variations associated with disease diagnosis. Each SNP represents a common genetic variant present in more than 1-5% of the population. Individually, SNP effects on disease risk are very modest, usually less than 20% increase in relative risk.
However, by aggregating the effects of disease-associated SNPs, polygenic risk models can categorize individuals along a spectrum of low to high inherited risk. Polygenic scores typically explain 7-12% of disease variance, though up to 25% for some cancers. The more powerful the original GWAS in terms of sample size, the better the polygenic score will be at predicting an individual’s predisposition.
Constructing Polygenic Scores
Various methods exist for constructing polygenic scores after identifying disease-associated SNPs through GWAS. Most commonly, a SNP effect size is multiplied by the number of risk alleles (0, 1 or 2) for that SNP in a given individual. These products are summed across all chosen SNPs to derive an overall polygenic risk score. SNPs strongly associated with disease receive more weight than weakly associated markers.
Rigorous validation in independent sample sets evaluates the predictive performance of polygenic scores. Optimal SNP inclusion thresholds are selected to maximize predictive ability. Polygenic models lose power with too few or too many SNPs included. Ideal thresholds retain SNPs explaining at least 0.01% of disease variance based on GWAS significance levels.
Applications and Limitations
Polygenic risk models are currently most advanced for coronary artery disease, breast and prostate cancer, type 2 diabetes and inflammatory bowel disease. Potential clinical applications include:
- Risk stratification to guide evidence-based screening recommendations beyond family history.
- Targeted prevention and lifestyle modification for individuals at elevated genetic risk.
- Informing reproductive decision-making and genetic counseling based on polygenic risk.
- Improving disease prediction, subtyping and prognosis when combined with clinical risk factors.
However, limitations and ethical concerns exist around polygenic score implementation:
- Most heritability remains unexplained. Adding more SNPs only incrementally improves prediction.
- Polygenic testing may prompt unnecessary interventions if clinical validity and utility are not adequately demonstrated.
- Possible psychological harm and discrimination from genetic risk probabilization.
- Unequal health benefits if not equitably implemented across populations.
While polygenic scores currently identify individuals with modestly increased or decreased disease risks, their predictive utility is anticipated to grow exponentially with million-person biobank efforts and whole-genome sequencing. Harnessing the full spectrum of genomic variation contributing to polygenic inheritance will enable more personalized risk assessment and clinical decision-making for complex chronic diseases.
Sources:
- Torkamani A, Wineinger NE, Topol EJ. The personal and clinical utility of polygenic risk scores. Nat Rev Genet. 2018 Sep;19(9):581-590.
- Lewis CM, Vassos E. Polygenic risk scores: from research tools to clinical instruments. Genome Med. 2020 May 6;12(1):44.
- Khera AV, Chaffin M, Zekavat SM, et al. Whole-genome sequencing to characterize monogenic and polygenic contributions in patients hospitalized with COVID-19. Nat Commun. 2021 Jan 20;12(1):536.
- Torkamani A, Erion G, Wang J, et al. An evaluation of polygenic risk scores for predicting breast cancer. Breast Cancer Res Treat. 2019 Apr;175(2):493-503.
- Mars N, Koskela JT, Ripatti P, Kiiskinen T TJ, Havulinna AS, Lindbohm JV, Ahola-Olli A, Kurki M, Karjalainen J, Palta P, FinnGen, Neale B, Daly M, Salomaa V, Palotie A, Collins F, Samani N, Ripatti S. Polygenic and clinical risk scores and their impact on age at onset and prediction of cardiometabolic diseases and common cancers. Nat Med. 2020 Nov;26(11):1660-1666.